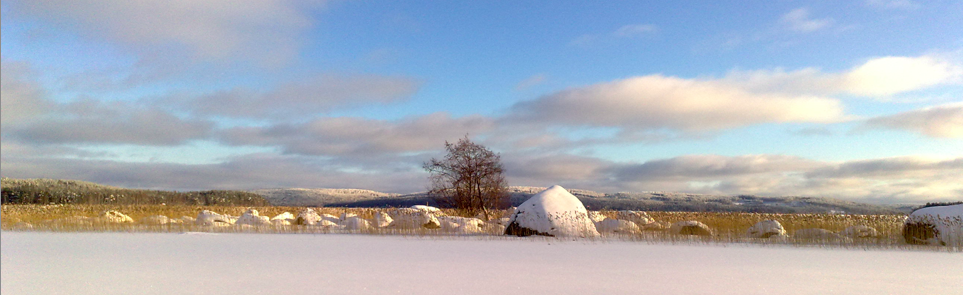
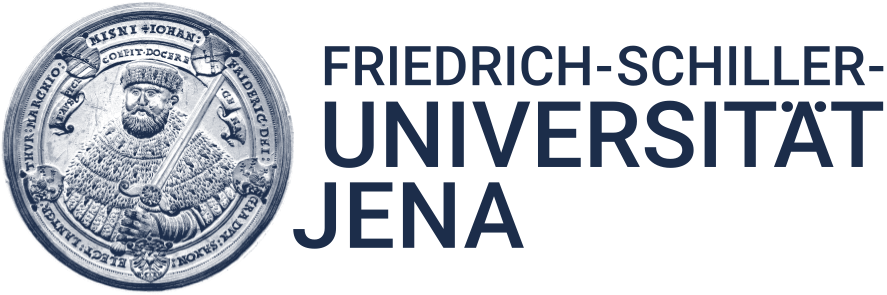
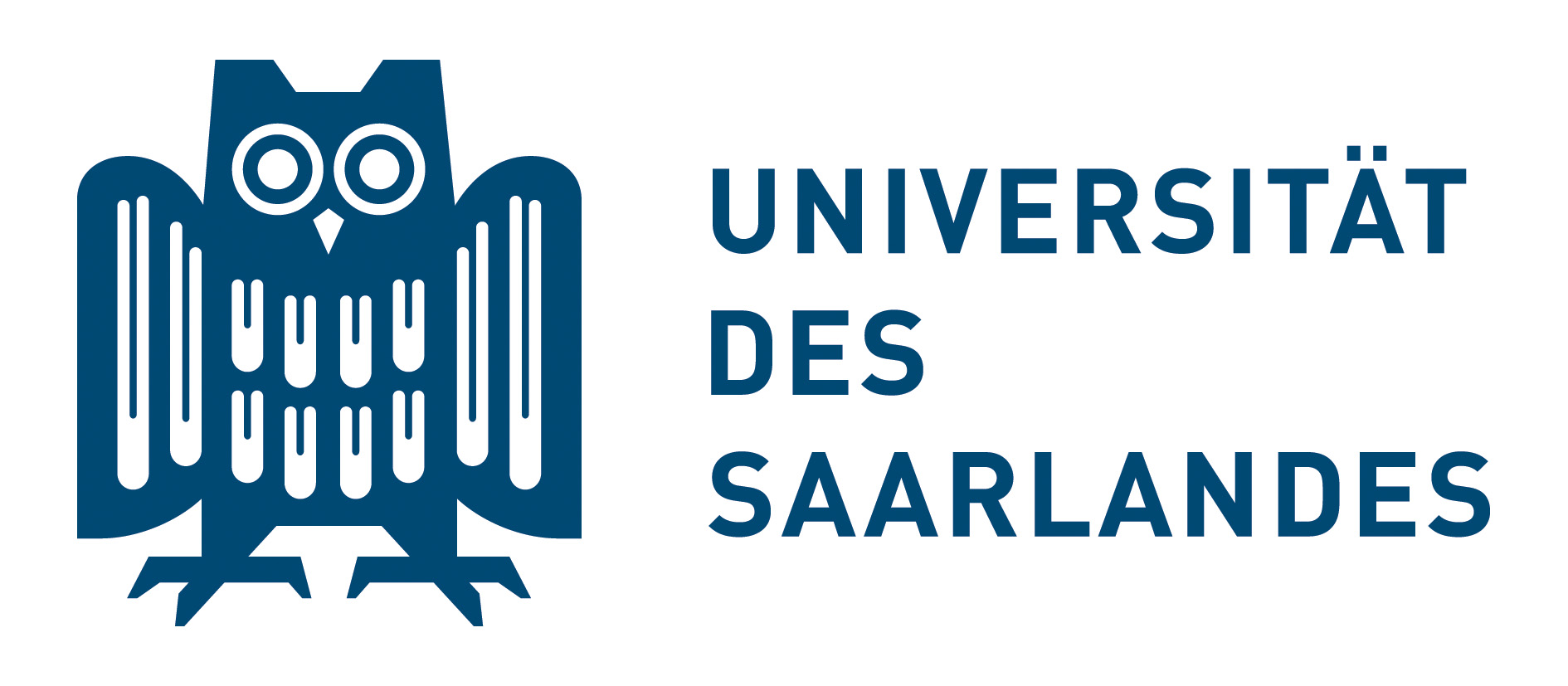
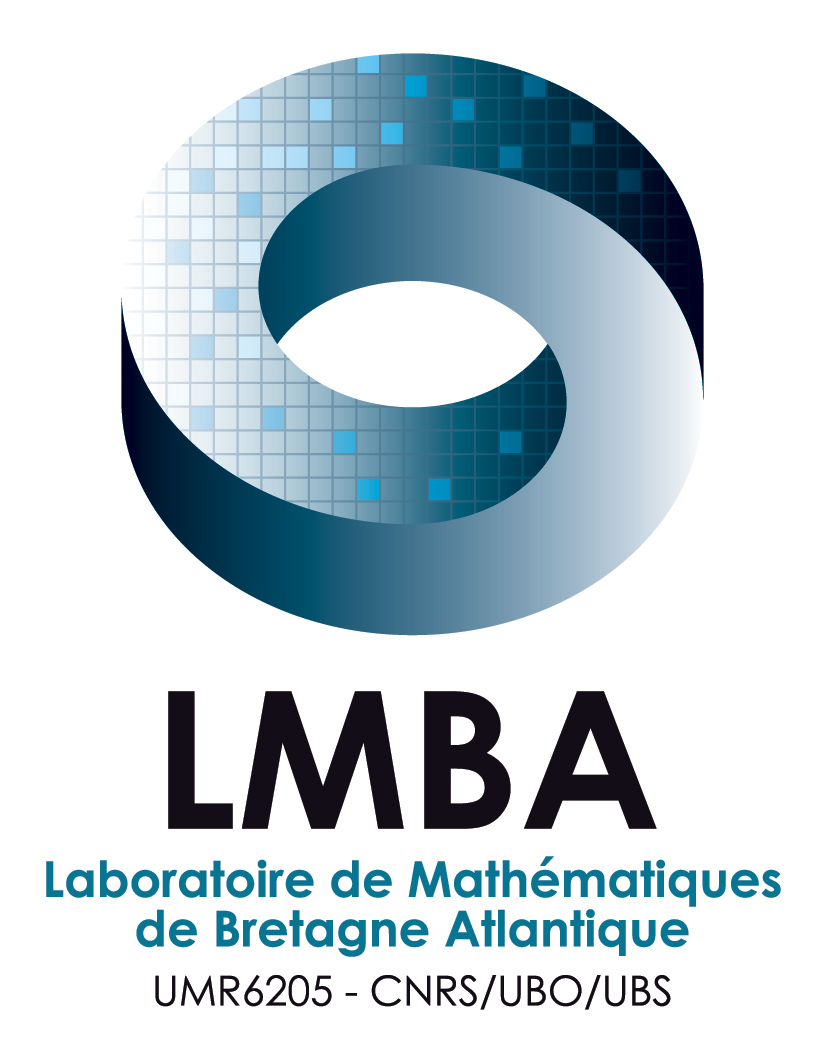
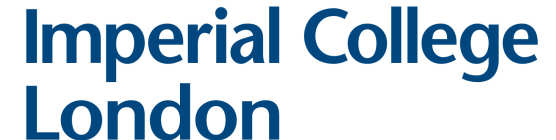
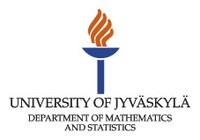
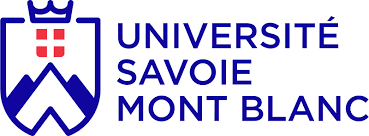
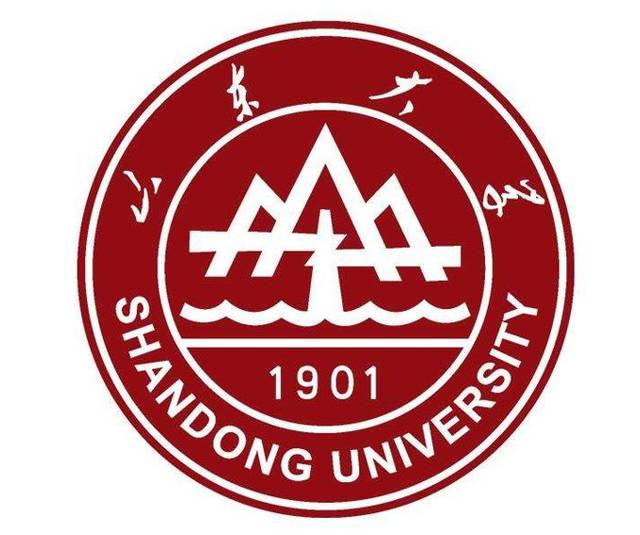
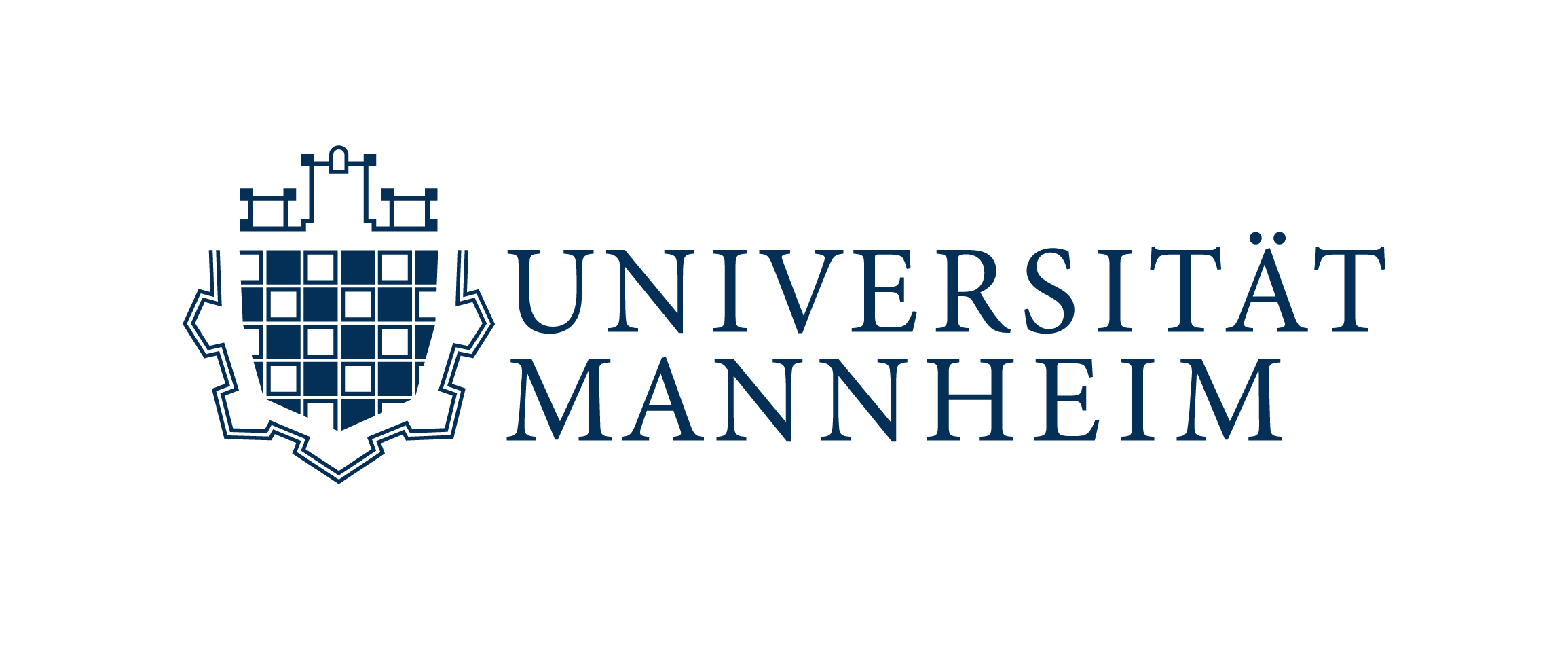
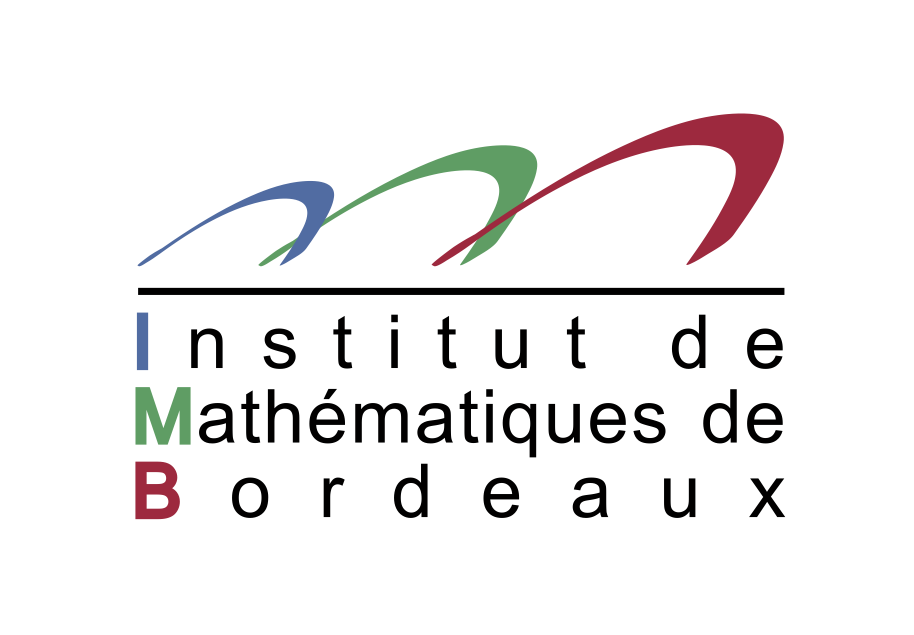
International Seminar on SDEs and Related Topics
- This online seminar takes place about every four weeks on Friday at
12:30 UTC | 11:30 UTC during European daylight saving times (ending October 27) |
---|
12:30 noon | 1:30 pm | 2:30 pm | 7:30 pm (8:30 pm after Oct 27) |
---|---|---|---|
London | Berlin, Paris | Helsinki | Beijing |
Zoom link Meeting ID: 618 9100 7917
No registration required. To get an e-mail reminder before each event write to sde-seminar[at]jyu.fi.
Organisers
- Stefan Ankirchner (FSU Jena, Germany)
- Christian Bender (Saarland University, Germany)
- Rainer Buckdahn (Universite de Bretagne Occidentale, France)
- Dan Crisan (Imperial College London, UK)
- Hannah Geiss (University of Jyväskylä, Finland)
- Stefan Geiss (University of Jyväskylä, Finland)
- Céline Labart (Université Savoie Mont-Blanc, France)
- Juan Li (Shandong University, China)
- Andreas Neuenkirch (University of Mannheim, Germany)
- Shige Peng (Shandong University, China)
- Adrien Richou (University of Bordeaux, France)
Schedule 2024 Autumn
Oct 18, 2024
Michael Röckner Poster:
(Bielefeld University, Germany)
Abstract:
In this talk we shall present the construction of a stochastic process, which is related to the parabolic
p-Laplace equation in the same way as Brownian motion is to the classical heat equation given by the
(2-) Laplacian.
Joint work with:
1) Viorel Barbu, Al.I. Cuza University and Octav Mayer Institute of Mathematics of
Romanian Academy, Iaşi, Romania
2) Marco Rehmeier, Faculty of Mathematics, Bielefeld University, Germany
References
[1] V. Barbu, M. Rehmeier, M. Röckner: arXiv:2409.18744
[2] V. Barbu, M. Röckner: Springer LN in Math. 2024
[3] M. Rehmeier, M. Röckner: arXiv:2212.12
Nov 15, 2024
Mathias Beiglböck Poster:
(Universität Wien, Austria)
Abstract: In classical optimal transport, the contributions of Benamou−Brenier and McCann regarding the time-dependent version of the problem
are cornerstones of the field and form the basis for a variety of applications in other mathematical areas.
Stretched Brownian motion provides an analogue for the martingale version of this problem. We provide a characterization in terms of gradients of convex
functions, similar to the characterization of optimizers in the classical transport problem for quadratic distance cost.
This is based on joint work with Julio Backhoff-Veraguas, Walter Schachermayer and Bertram Tschiderer.
Dec 13, 2024
Arnulf Jentzen Poster:
(The Chinese University of Hong Kong, Shenzhen (CUHK-Shenzhen), China and University of Münster, Germany)
Abstract: Partial differential equations (PDEs) are among the most universal tools used in modelling
problems in nature and man-made complex systems.
Nearly all traditional approximation algorithms for
PDEs in the literature suffer from the so-called "curse of dimensionality" in the sense that the number of required
computational operations of the approximation algorithm to achieve a given approximation accuracy grows
exponentially in the dimension of the considered PDE. With such algorithms it is impossible to approximately compute
solutions of high-dimensional PDEs even when the fastest currently available computers are used.
In the specific situation of
linear parabolic PDEs and approximations at a fixed space-time point, the curse of dimensionality of deterministic methods can be
overcome by means of Monte Carlo approximation algorithms and the Feynman-Kac formula.
In this talk we show that deep neural
networks (DNNs) have the fundamental property to be able to approximate solutions of semilinear PDEs with Lipschitz nonlinearities
with the number of real parameters of the approximating DNN growing at most polynomially in, both, the reciprocal of the prescribed
approximation accuracy and the PDE dimension. Our arguments are strongly based on suitable nonlinear Monte Carlo methods for such PDEs.
In the second part of the talk we present some recent mathematical results for stochastic gradient descent (SGD) optimization methods for the
training of DNNs such as the popular Adam optimizer.
Schedule 2025 Spring
Jan 17, 2025
Jean-François Chassagneux(ENSAE Paris, France)
Abstract: Under some confluence assumption, it is known that the stationary distribution of a McKean-Vlasov SDE is the limit of the empirical measure of
its associated self-interacting diffusion.
Our numerical method consists in introducing the Euler scheme with decreasing step size of this self-interacting diffusion and seeing its empirical measure
as the approximation of the stationary distribution of the original McKean-Vlasov SDEs.
This simple approach is successful (under some reasonable assumptions...) as we are able to prove convergence with a rate for the Wasserstein
distance between the two measures both in the L2 and almost sure sense.
In this talk, I will first explain the rationale behind this approach and then I will discuss the various convergence results we have obtained so far.
This is a joint work with G. Pagès (Sorbonne Université).
Feb 21, 2025
Gianmario Tessitore(UNIMIB University of Milano-Bicocca, Italy)
Abstract: This talk is devoted to the optimal control of a system with two time-scales, in a regime when the limit equation is not of averaging
type but, in the spirit of Wong-Zakai principle, it is a stochastic differential equation for the slow variable, with noise emerging from the fast one.
It proves that it is possible to control the slow variable by acting only on the fast scales. The concrete problem, of interest for climate research,
is embedded into an abstract framework in Hilbert spaces, with a stochastic process driven by an approximation of a given noise. The principle
presented here is that convergence of the uncontrolled problem is sufficient for convergence of both the optimal costs and the optimal controls.
This target is reached using Girsanov transform and the representation of the optimal cost and the optimal controls using a Forward Backward System.
This is joint work with F. Flandoli - Scuola Normale Superiore, G. Guatteri - Politecnico di Milano and U. Pappalettera - Universitat Bielefeld.
Mar 14, 2025
Apr 11, 2025
Arnaud Debussche(ENS Rennes, France)
May 09, 2025
Emmanuel Gobet(CMAP-Ecole Polytechnique, France)
Jun 06, 2025
Contact and links
-
Stefan Geiss: stefan.geiss[at]jyu.fi or stefanfriedrich.geiss[at]gmail.com
-
The online seminar is hosted by the Department of Mathematics
and Statistics (University of Jyväskylä) and supported by the journal
Probability, Uncertainty and Quantitative Risk.